EXplAIn
Facts
Academic Partner: Jönköping University
Financier: The Swedish Research Council
Duration of the project: 2019 - 2022
Project manager: Maria Riveiro, Professor
Evaluation of Explainable Artificial Intelligence (EXplAIn) tackles the challenge of evaluating users interacting with ML-systems, aiming at developing a generic and integrative framework for evaluating human ML-system collaboration.
Introduction
We live in a fascinating big data world, full of challenges, but also possibilities. Over the course of the next 20 years more will change around the way we do our daily activities than it has happened in the last 2000; we are entering an augmented age, where our natural capabilities are being augmented by AI technologies that help us think, make and be connected.
However, understanding how people interact with Machine Learning (ML) technologies is critical to design and evaluate systems that people can use effectively. Unfortunately, ML is often conceived in an impersonal way and ML algorithms are often perceived as black-boxes, which hinders their use and their full exploitation in our daily activities.
EXPLAIN tackles the challenge of evaluating users interacting with ML-systems. We argue that to be able to evaluate these interactive processes, we need to include theoretical principles from Cognitive Science that account for human preconceptions about systems' inner workings and behavior.
Expected outcomes
We develop a generic and integrative framework for evaluating human ML-system collaboration, combining traditional methods from ML and HCI with principles from Cognitive theories rarely considered in this interdisciplinary field.
The overall goal is to contribute to explaining our interactions with AI-technologies, moving forward towards more usable AI for augmented intelligence.
News
- The project XPECT (How to Tailor Explanations from AI Systems to Users' Expectations, funded by the Swedish Research Council (Vetenskapsrådet) will start in the fall 2023!
- Maria Riveiro is now a part of AcademiaNet
External link, opens in new window. (by invitation only, invited by Vetenskapsrådet). AcademiaNet is an expert database for outstanding female academics. Webpage
External link, opens in new window..
- Maria Riveiro participated in Dagstuhl Seminar 22351, second part of
Interactive Visualization for Fostering Trust in MLExternal link, opens in new window. (Aug 28 – Sep 02, 2022)
- Maria Riveiro participated in Dagstuhl Seminar 22331 Visualization and Decision Making Design Under Uncertainty
External link, opens in new window. (Aug 15 – Aug 19, 2022)
- Jönköpings-Posten article on Maria's AI-research and JAIL here
External link, opens in new window. (1st Nov 2021)
- Maria Riveiro wil join the Dagstuhl Seminar "Interactive Visualization for Fostering Trust in AI
External link, opens in new window." in September 2020, Schloss Dagstuhl, Wadern, Germany.
- Maria Riveiro participated in the Dagstuhl Seminar “Machine Learning Meets Visualization to make AI Interpretable
External link, opens in new window.” in November 2019, Schloss Dagstuhl, Wadern, Germany. Summary report
External link, opens in new window..
Publications
- Riveiro, M. (2023). Expectations, trust, and evaluation Dagstuhl Reports, 12(8), 109. More information
- Riveiro, M. (2023). A design theory for uncertainty visualization? Dagstuhl Reports, 12(8), 12-13. More information
- Riveiro, M. and Thill, S. (2022). The challenges of providing explanations of AI systems when they do not behave like users expect. In Proceedings of the 30th ACM Conference on User Modeling, Adaptation and Personalization (UMAP ’22), July 4–7, 2022, Barcelona, Spain. ACM, New York, NY, USA, 11 pages. https://doi.org/10.1145/3503252.3531306
- Beauxis-Aussalet, E., Behrisch, M., Borgo, R., Chau, D. H., Collins, C., Ebert, D., El-Assady, M., Endert, A., Keim, D. A., Kohlhammer, J., Oelke, D., Peltonen, J., Riveiro, M., Schreck, T., Strobelt, H. and van Wijk, J. J. "The Role of Interactive Visualization in Fostering Trust in AI," in IEEE Computer Graphics and Applications, vol. 41, no. 6, pp. 7-12, 1 Nov.-Dec. 2021, doi: 10.1109/MCG.2021.3107875.
- 2nd Place Blue Sky Paper Award (including 750 USD travel grant), Björn Schuller, Tuomas Virtanen, Maria Riviero, Georgios Rizos, Jing Han, Annamaria Mesaros, Konstantinos Drosos, “Towards Sonification in Multimodal and User-friendly Explainable Artificial Intelligence
External link, opens in new window.”, 23rd ACM Int. Conf. on Multimodal Interaction (ICMI 2021), ACM, Montreal, Canada, 18-22.10.2021.
- Riveiro, M., & Thill, S. (2021). “That's (not) the output I expected!” On the role of end user expectations in creating explanations of AI systems
External link, opens in new window.. Artificial Intelligence, Volume 298, 103507.
- Riveiro, M. (2020). Explainable AI for maritime anomaly detection and autonomous driving. Dagstuhl Reports, 9(11), 29-30.
- Thill, S., Riveiro, M. (2019). Memento hominibus: on the fundamental role of end users in real-world interactions with neuromorphic systems. Robust Artificial Intelligence for Neurorobotics, 26 – 28 August 2019, Edinburgh, Scotland.
Financiers
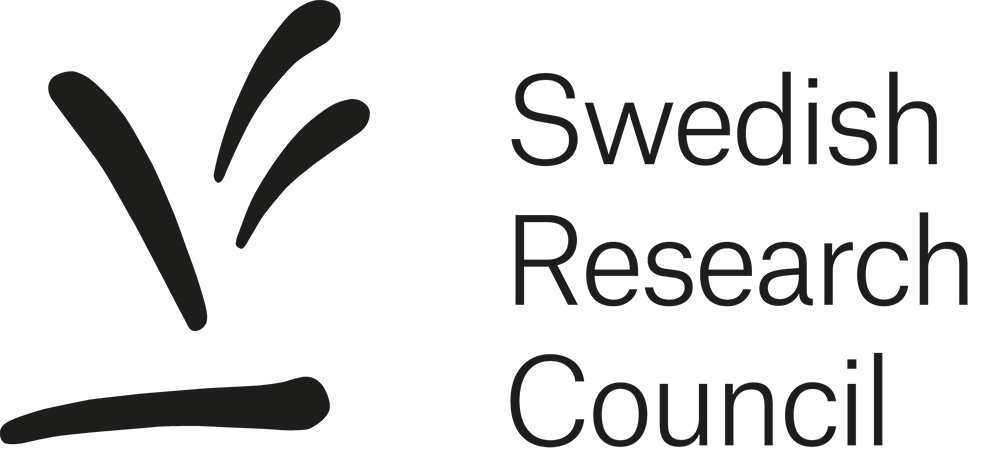
For more information
- Professor Computer Science
- School of Engineering
- maria.riveiro@ju.se
- +46 36-10 1578