Thermal Estimation of Electric Machine Rotor using ML for Volvo Cars
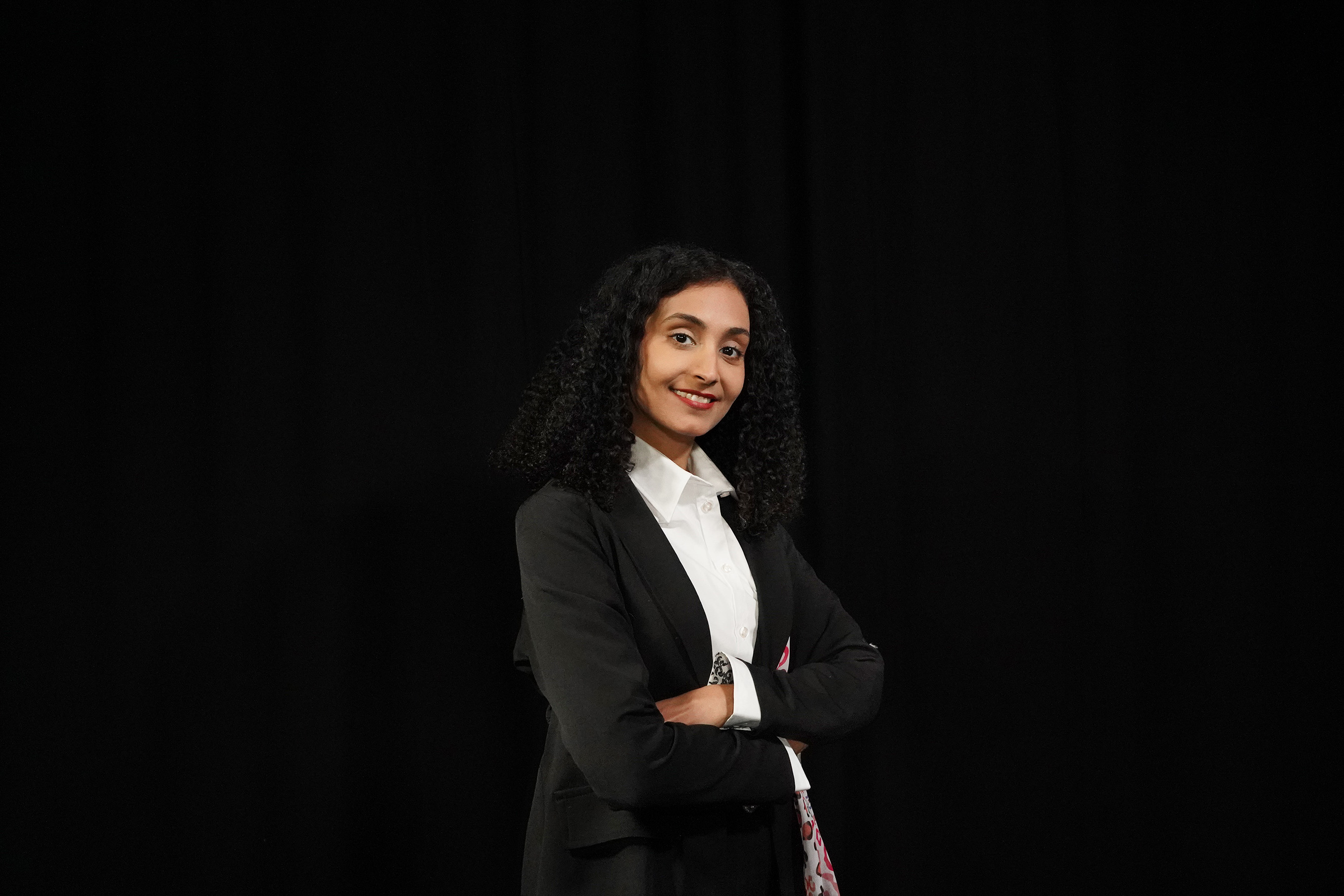
Martin Hanke
LinkedIn Länk till annan webbplats, öppnas i nytt fönster.
Nadera Najib Al-Areqi
This thesis explores the application of machine learning to predict rotor temperatures in induction motors, aiming to enhance the safety, efficiency, and longevity of electric machines, with a focus on models developed in collaboration with Volvo Cars.
The thesis "Thermal Estimation of Electric Machine Rotor using ML for Volvo Cars" investigates machine learning (ML) techniques to predict rotor temperature in induction motors, crucial for enhancing safety, efficiency, and longevity of electric machines. Three neural network models were developed and evaluated: Multilayer Perceptron (MLP), Thermal Neural Network (TNN), and 1-D Convolutional Neural Network (1-D CNN). The study utilized a dataset of over 2.8 million data points, covering a wide range of operational scenarios. The TNN model, which incorporates heat-transfer principles, outperformed other models in accuracy and reliability, achieving mean squared errors of 4.2 and 23.3 in different driving scenarios.
Experimental results demonstrated that ML models provide a more adaptable and precise approach to temperature estimation compared to traditional deterministic models. Particularly, including motor housing temperature data significantly improved prediction accuracy, suggesting potential cost savings by eliminating the need for stator temperature sensors. The research emphasizes the superiority of ML models for predicting electric motor temperatures, with the TNN model showing the most promise for real-time motor control system integration.